“There were 5 exabytes of information created between the dawn of civilization through 2003, but that much information is now created every two days.” – Eric Schmidt, Google
Human beings are now, on a semi-daily basis, generating and collecting data that equals the volume of the total collective knowledge of our species till around the turn of the millennium – somewhere in the range of 3.5 quintillion bytes.
In mathematical language, that’s 18 zeroes! This is a mind-bogglingly large number, and it leads naturally to the question…
What are we doing with all of this data? Are we using it effectively, and sharing it efficiently? Is the explosion of data storage, collection and analysis infrastructure in the last 10-15 years warranted?
Our world is so fundamentally reliant on data collection and analysis, that data in and of itself has become an incredibly important product. In this piece, we’ll talk about what data products are, the current situation of data products and share our perspectives around how this technology might evolve.
How Much Is Too Much?
In the past few years, it is like the data collection Pandora’s Box has been opened. Organizations large and small have been investing massively into data collection and manipulation, and the number of entities joining the movement is growing by the day.
These past few years have also introduced us to a wide variety of data infrastructure technologies. Businesses are now creating data artifacts at a rapid pace due to the widespread availability of data tools.
However, we’ve seen that the value and output isn’t necessarily proportional to the investment. While making data artifacts might have become easier, the actual TCO and cost of managing them through their lifecycle has increased dramatically.
One of the main reasons for the current state of low ROI and mismatched value perception in the data product space is that companies lost track of why they were building these complex data technologies in the first place. As a result, driving value through actual business metrics such as customer churn, improving the customer experience, reduction of risk, and many more, have been ignored.
To bring things back around, organizations must go back to first principles and turn their focus to the fundamental purpose of any data collection infrastructure – to fuel processes that have measurable outcomes and move the organizational needle forward.
What are data products?
The system or entity which bridges the infrastructure, data and end-user value is what we call a Data Product. This could refer to a BI (Business Intelligence) system, simple tables, derived datasets, machine models, reports, dashboards, and more. The nature of a data product could be at multiple levels of granularity. It could be a standalone product or a much more complex platform with a collection of data products embedded into it.
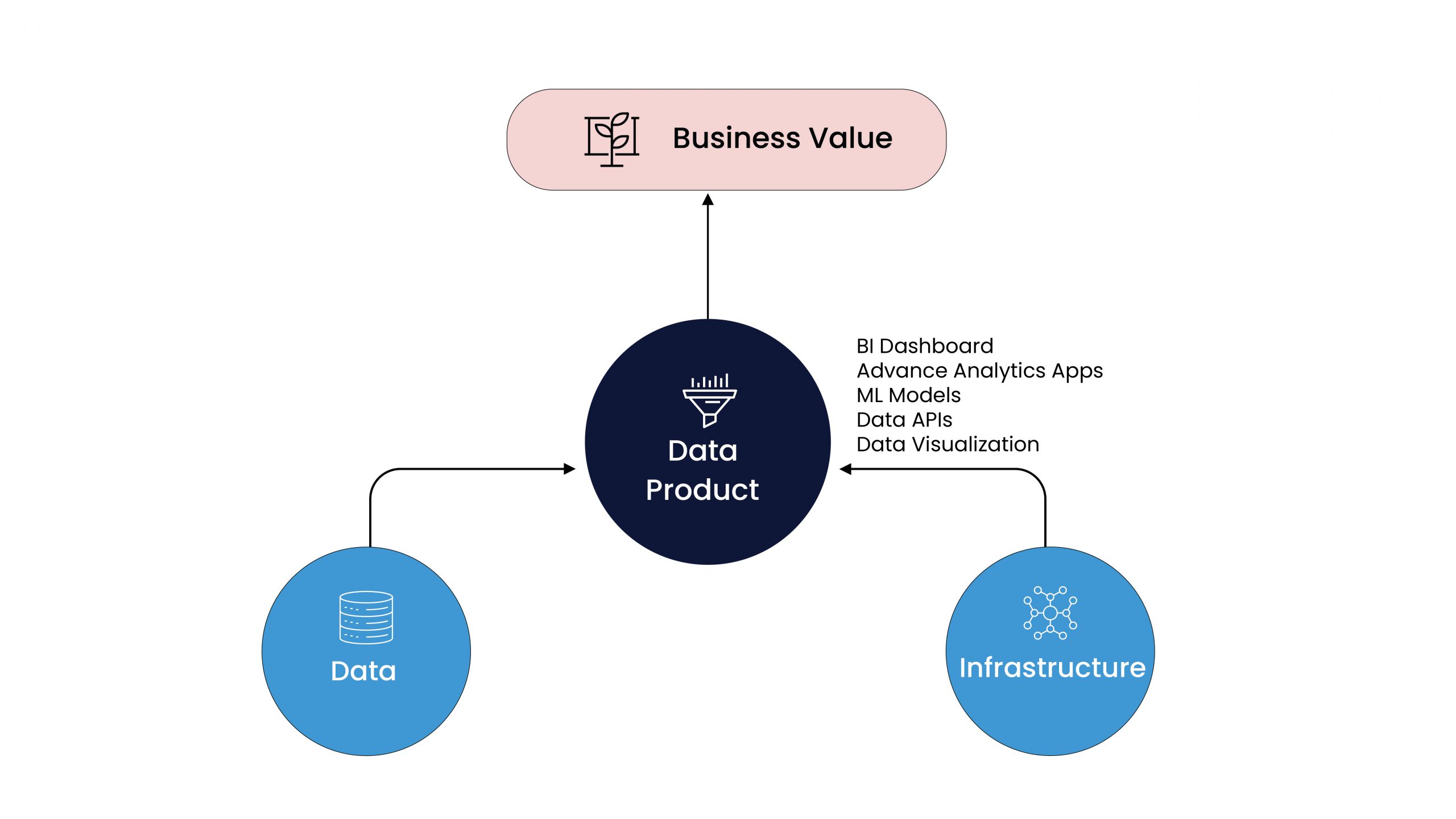
A data product bridges the infrastructure, data, and end-user or business value.
But why treat data as a product in the first place?
Once data is productized, it automatically creates an internal customer, an end user and a set of well-defined boundaries, an SLA (service-level agreement) and deliverables for the data product. This internal customer will have a set of requirements and constraints that the product must deliver on for its end users. The end user will have a set of expectations for correctness, visualization, detail level, auditability, legality and safety.
This type of systemization makes it possible to create a series of standards and thresholds around data artefacts, enabling data products to be quantified and usable repeatedly to create reliable, well-defined outcomes.
Consider the example of Gasoline – a compound derived from crude oil through the process of distillation. Before gasoline reaches its final form, there are a lot of compounds, catalysts and substances that must be present in a specific quantity at a specific temperature. Without standardization and management of each “product” within the larger system that creates gasoline, we would notice irregularities in the quality of fuel we use in our vehicles.
What are the current challenges with data products?
A lot of the current conversation around data products focuses on the specific technical mechanisms – which API, which Docker, which modelling strategy etc. In our opinion, we need to look elsewhere to make data products better.
Right now, data products are in a quasi no man’s land. They don’t belong to any one organizational division (software engineering/data engineering/analytics), which makes them difficult to manage internally. If we go function by function, the product managers are probably the most aligned to be answerable for data products. However, product managers are usually tasked with making the end-user experience of the app or product – they aren’t data product management specialists.
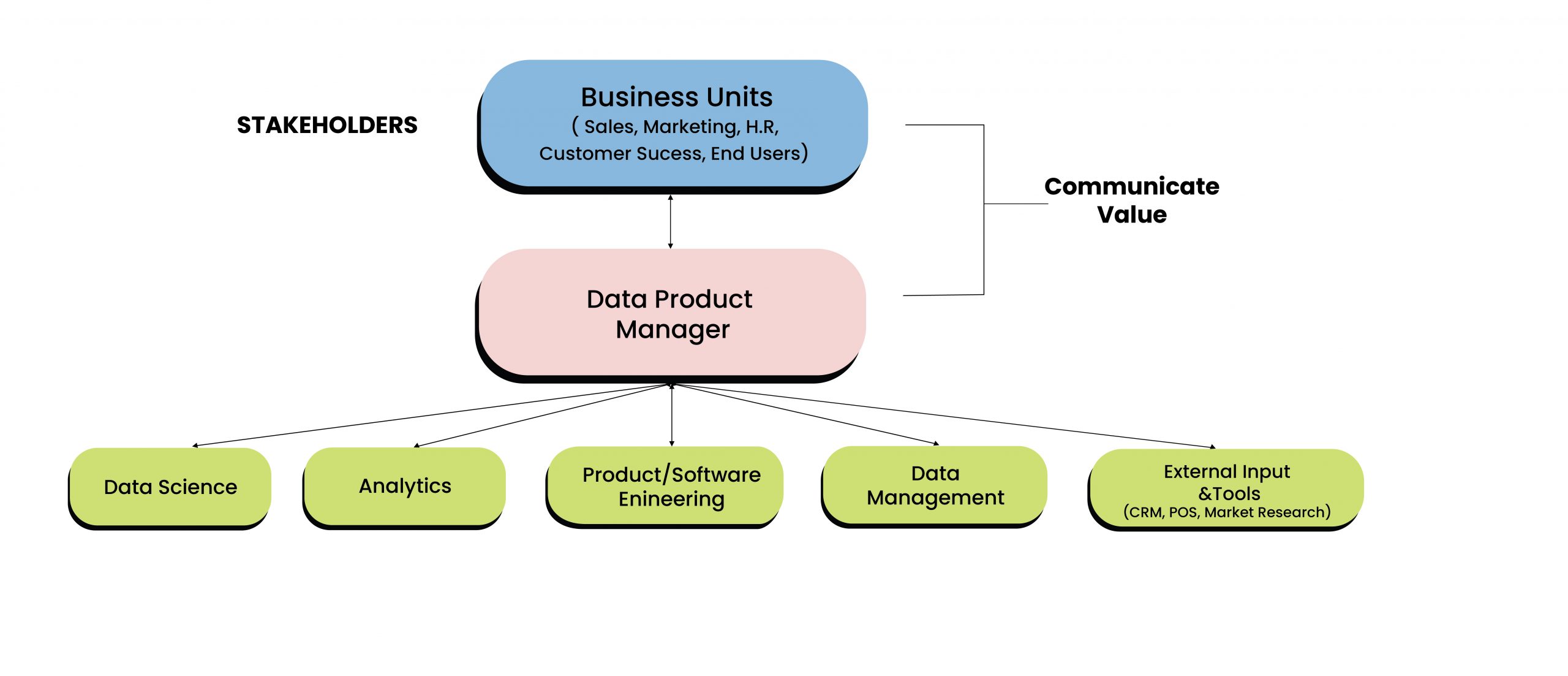
The role of a data product management specialist
Since there aren’t many specialist data product managers and platform product managers, it is difficult to balance the tech and the end user experience to create data products which drive business decisions. As a result, the universe of data products as a whole has remained underdeveloped, and there is a ton of unexplored potential value.
Building Better Data Products
Now that we’ve touched upon the constraints and desired outcomes for data products, let’s look at two important implications that come along with building them.
- If a product exists, there is an implicit expectation of touch and feel. So, if the product exists, then users should be able to discover it, look at it and evaluate it. So, these products might exist within a marketplace or some kind of repository or active workshop within the organization. Whichever form these data products take, they must be discoverable to a consumer.
- With products, there is automatically an economical component to their continued existence. For example, the product may only be financially viable under certain constraints. As a result, most data products are highly specific to individual organizations. There may be situations where the product needs to be customized to be usable by a mass audience. For example, Retool and Airtable are data product platforms where individual data products can be discovered, shared and reused. We’re still in the early stages of data products though, because we expect future data products to be richer and more interactive.
It’s not like we don’t have data products now. As mentioned earlier, a simple table, or a BI system can be considered a data product as well. However, the challenge right now is that simple apps aren’t able to deal with the increasingly complex decision-making they need to perform. Our inputs are getting more complex, data is more sophisticated and the questions we are asking are much more nuanced. As a result, we expect that a whole new generation of more complex apps is on the horizon.
The Economics of Better Data
We foresee that data products will be mass customized because each business unit within an organization will have different use case requirements. For example, a finance entity which requires foreign exchange rate predictions will have completely different requirements than someone in the operations department.
All of this mass customization is not going to be cheap or easy. It will also be an iterative process that will evolve over time. In this new world, things like app platforms will have clear economics. Organizations can look at individual apps and perform a cost-benefit analysis to select compatible ones.
One of the challenges with evaluating the financial feasibility of data products today is that all of these costs are part of one big data engineering bucket. These costs have significant linkage to the outcome, and this impact isn’t present in the current budgeting process.
Another challenge with current budgeting is that it optimizes for development cost and not operational cost (what the products will cost over their lifetime due to changes and evolution). All of the time that will be spent debugging, iterating and improving the data products needs to be accounted for.
Our estimate is that the operational costs are 10x that of the development costs which is why the total cost of ownership (TCO) calculations are inaccurate
A realistic TCO will account for the full life cycle cost including the cost of terminating the product itself.
Overall, having a comprehensive data product ecosystem will make the creation and sustenance of better data products financially viable and sustainable over the long run.
Data Products Need to Be Nimble, Malleable
Data products don’t operate with a specification-based approach. What does that mean? Well, end users don’t actually know what these data products are – they only have an idea or a perception of a specific version of a specific data product.
Data products will need to go through an evolutionary process. As a result, the technology, the products, the platform and our very approach itself must be nimble. It should allow organizations that use the products to evolve and customize them, because with each iteration the product gets closer to the optimal use cases and therefore becomes more valuable to the organization.
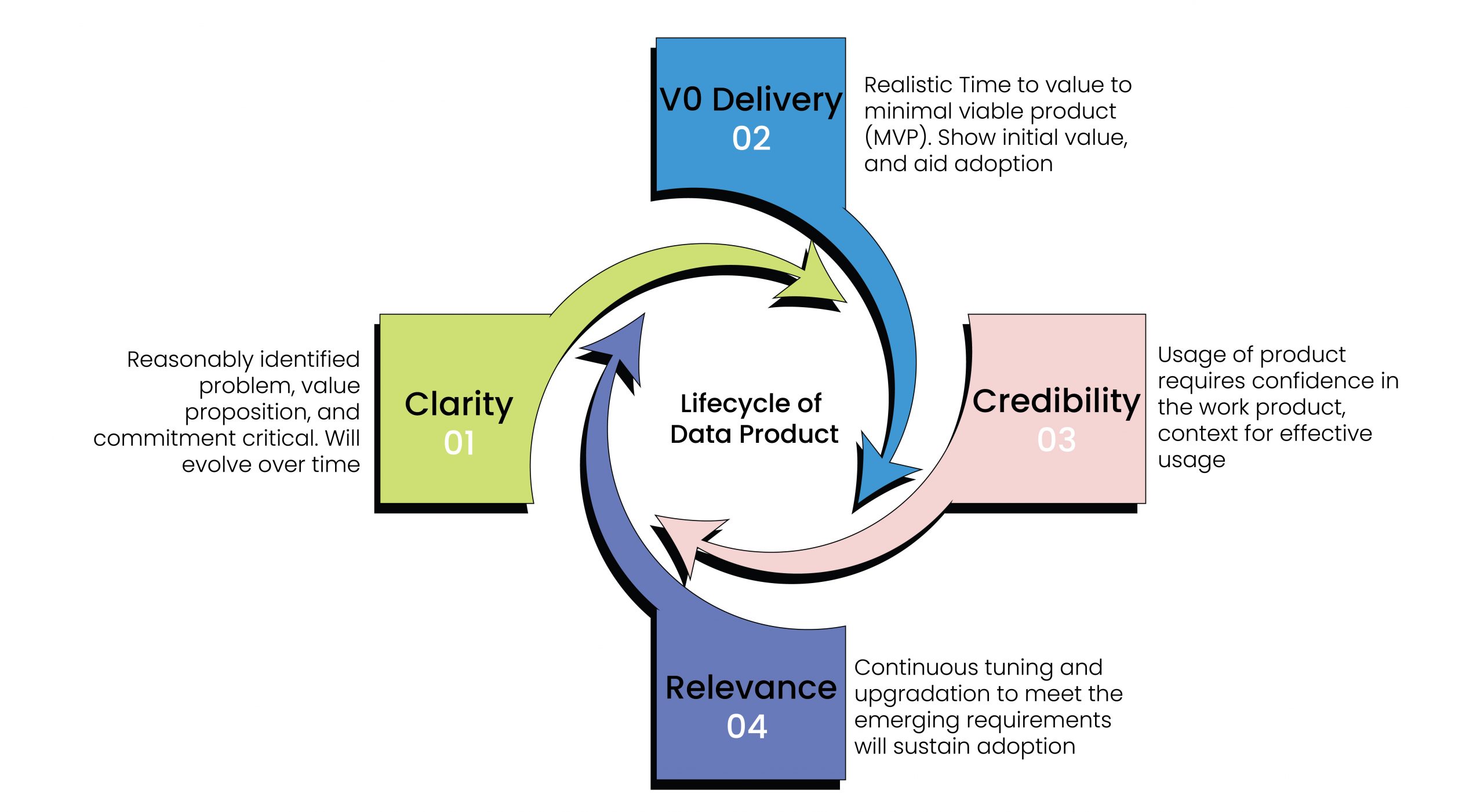
The iterative and evolutionary process of building data products
What’s next?
Currently, the way we’re all looking at data apps is like a thin layer on top of existing data warehouses. But the way we view data products at Scribble Data, we believe they have much deeper value propositions. You can think of them as thick apps – embedding a lot of the domain knowledge and workflow understanding (with the storage layer in the warehouse being merely incidental). So, data apps themselves will come in various forms – thick, very thin, and medium.
If our outlook towards data products remains nimble, we will see rapid proliferation of several different types of data products – from something as simple as a table to something as complex as risk models for the manufacturing industry. There will need to be a life cycle for all of these apps and economics that support a bunch of products, tools and technologies to help compute value and manage the process.
We’re still in the early days of data productization, but at Scribble Data, we’ve helped companies build hundreds of rapid data products. Watch this space to learn more about data products.
Reach out to us to learn more about how Enrich, our full-stack platform can help you go from raw data to an operationalized data product 5x faster!