Scene 1: Picture waking up to the soft strumming of the acoustic guitar on Bon Iver’s “Holocene”, a song recommendation from Spotify based on your recent obsession with indie folk.
Scene 2: As you sip your morning coffee, you scroll through your Amazon app, noticing a recommendation for a book on “Modern Folklore and Music.” Intrigued, you read the reviews, and with a single tap, it’s on its way to your doorstep.
Scene 3: Later in the day, while working on a project, Spotify’s algorithm seamlessly transitions your playlist to instrumental tracks, recognizing your need for focus. During your lunch break, Amazon pings you with a deal on a new coffee blend, remembering your purchase of a French press last month.
Scene 4: As the day winds down, you’re back on Spotify, this time exploring a playlist titled “Songs to Unwind.” Simultaneously, Amazon suggests a set of ambient lights, perfect for setting a relaxing mood in your room.
The seamless integration of multiple facets from your daily preferences, habits, and suggestions isn’t a mere coincidence. It’s the result of intricate data systems working behind the scenes, understanding you, predicting your needs, and enhancing your experiences. Much like the threads of a woven fabric, every piece of data, every logged preference, every recorded click, is interconnected, creating a personalized tapestry of your digital life.
This is the magic of data fabrics. It’s not just about storing information; it’s about interconnecting data from various sources, making it accessible, and using it to enhance user experiences. Just as Amazon and Spotify seem to “know” you, businesses are harnessing the power of data fabric architectures to create holistic, integrated, and responsive systems that cater to individual needs.
What is a Data Fabric?
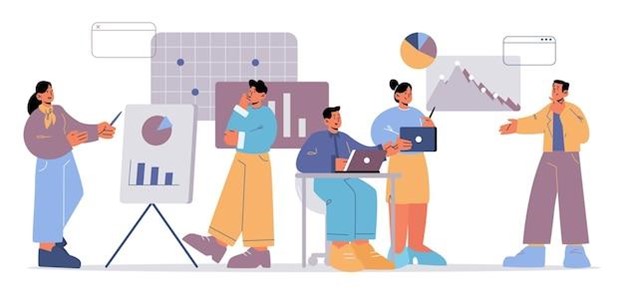
Image Source: freepik
Data Fabric is not just another data management tool; it’s an architecture and a set of data services that provide consistent capabilities across a range of endpoints spanning on-premises and multiple cloud environments. In simpler terms, think of it as the thread that weaves together various data sources, much like the threads in a fabric. It ensures seamless data integration, making data accessible, understandable, and usable across the organization.
In the vast expanse of the digital age, data has emerged as the lifeblood of modern enterprises. But to truly understand the significance of data fabrics, we must first embark on a journey through the evolution of data management.
The Dawn of Data Management
In the early days of computing, data was stored in hierarchical databases. These were rigid structures, much like a family tree, where data was organized in a top-down manner. This system worked well for straightforward tasks but was ill-equipped to handle the complexities of the burgeoning digital landscape.
As businesses grew and diversified, the need for a more flexible system became apparent. The relational databases model, pioneered by Dr. Edgar F. Codd in the 1970s, allowed data to be stored in tables, making it easier to establish relationships between different data sets. This was a game-changer, enabling businesses to query data in unprecedented ways and extract valuable insights.
Fast forward to the 21st century, and the data landscape underwent another seismic shift. The advent of the internet and the proliferation of devices led to an explosion in data volume, variety, and velocity. Traditional databases were overwhelmed. New frameworks, like Hadoop, emerged to process and store this avalanche of information.
The Emergence of the Postmodern Data Stack
The modern data stack, while revolutionary, faced challenges. It brought about a significant skills gap, steep learning curves, and complexities due to excessive accessibility.
The postmodern data stack offers a more agile approach, emphasizing machine learning at a reasonable scale. This new paradigm allows businesses to quickly build numerous machine learning models, optimizing a variety of operations.
Data fabric architectures serve a dual purpose. They aid operational use cases, ensuring smooth daily business operations, and support analytical use cases, helping businesses derive insights, predict market trends, understand customer behavior, and offer tailored services.
Organizations today pull data from diverse sources, from traditional databases to cloud platforms, IoT devices, and third-party APIs. Data fabric architectures ensure seamless integration of these sources.
In essence, data fabrics are the next evolutionary step in data management, ensuring that businesses can harness the full power of their data, no matter where it resides.
Key Components of Data Fabric Architectures
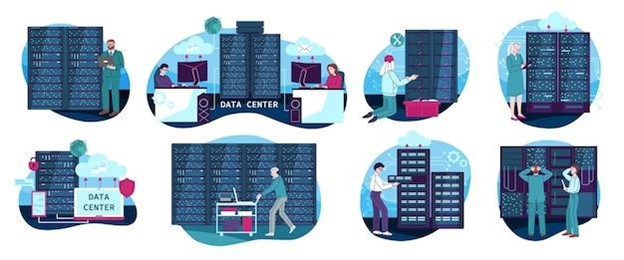
Image Source: freepik
In our journey through the realm of data fabrics, it’s essential to understand their foundational elements. These components are the pillars that uphold the intricate architecture, ensuring it functions seamlessly and efficiently.
- Active Metadata: Metadata, traditionally, has been like a label on a box, giving you a brief idea of what’s inside. But in the world of data fabrics, metadata is no longer just a static label. It’s dynamic, evolving, and self-updating. Imagine if the label on the box could change based on what you add or remove from the box, giving you real-time updates. That’s Active Metadata for you. It captures changes, learns from new data patterns, and adapts, ensuring that the data fabric remains relevant and up to date. This dynamic engagement allows for a more responsive data management system, adapting in real time to the needs of the business.
- Augmented Data Catalog: This component connects data assets with organizational terminology. By employing machine learning, it graphically displays metadata, forming the business semantic layer for the data fabric.
- Persistence Layer: A dynamic storage component, it accommodates data across a spectrum of relational and non-relational models based on specific use cases.
- Knowledge Graphs: Think of Knowledge Graphs as a detailed map of a bustling city. Just as a map shows you how different parts of a city connect; Knowledge Graphs map out the intricate web of relationships between different data entities. But it’s not just about connections; it’s about understanding the context behind them. For instance, knowing two places are connected by a road is useful, but understanding that it’s a one-way street during certain hours is invaluable. Similarly, Knowledge Graphs provide a holistic, contextual view of data interconnections, enabling businesses to derive meaningful insights.
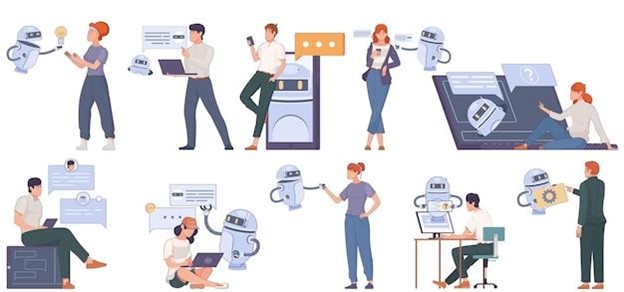
Image Source: freepik
- Semantics: In a world where the same word can have multiple meanings, ensuring consistent interpretation is crucial. Take the word “bank,” for instance. It could mean a financial institution or the side of a river. Semantics is all about ensuring that data, irrespective of its source, is interpreted in a consistent manner. It’s like having a universal translator that ensures everyone, or in this case, every system, speaks the same language, leading to more accurate insights and decision-making.
- Machine Learning and AI: Navigating the vast ocean of data manually is practically impossible. Machine Learning and AI make this task not just feasible but efficient. These technologies can predict data patterns, automate routine tasks, and even detect anomalies. For instance, if a business usually sees a certain volume of transactions, and suddenly there’s a spike, AI can flag this as an anomaly, prompting further investigation. This ensures that the data fabric remains robust, reliable, and ahead of potential issues.
- Insights and Recommendations Engine: This engine crafts reliable data pipelines for both operational and analytical use cases, ensuring data is processed efficiently.
- Data Preparation and Data Delivery Layer: This layer retrieves and delivers data from any source to any target, ensuring seamless data flow across the fabric.
- Orchestration and Data Ops: Coordinating the end-to-end workflow with data, this component determines how pipelines run and controls the data they generate.
- Data Services: While building a house, you would need a foundation, walls, roof, plumbing, and electricals. Similarly, the data fabric architecture is supported by a suite of services that form its foundation and structure. From discovering new data sources to ensuring that the data is secure and protected, data services are the building blocks of the data fabric architecture. They ensure that data is not just integrated but is also accessible, understandable, and usable across the organization.
Having delved into the core components of data fabrics, it’s evident how each element plays a pivotal role in creating a cohesive, integrated, and efficient data management system. In the next section, we’ll explore the tangible benefits businesses can reap by adopting this revolutionary approach to data management.
Benefits of Adopting Data Fabric Architectures
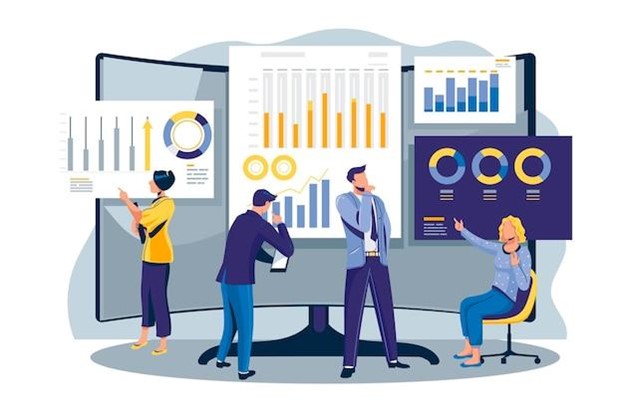
Image Source: freepik
In today’s digital age, where data is the cornerstone of decision-making, businesses are constantly seeking ways to harness its full potential. The data fabric architecture emerges as a solution that offers a suite of benefits tailored to meet the evolving needs of modern enterprises:
- Improved Data Integration: One of the primary advantages of data fabrics is their ability to integrate data seamlessly across diverse sources and platforms. Whether it’s data from on-premises databases, cloud platforms, IoT devices, or third-party APIs, data fabrics ensure that all data is harmonized and accessible. This integration is crucial in sectors like Fintech, where real-time data-driven insights can make the difference between a successful transaction and a missed opportunity.
- Enhanced Data Discoverability: With the vast amounts of data generated daily, finding relevant information can be akin to searching for a needle in a haystack. Data fabric architectures address this challenge by making data easily accessible and understandable. Through features like active metadata and knowledge graphs, users can quickly locate and understand the data they need, streamlining decision-making processes.
- Scalability: As businesses grow, so does the volume and complexity of their data. Traditional data management systems often struggle to scale without compromising performance. With the flexible data fabric architecture, businesses can adapt to increasing data volumes without any degradation in performance. This scalability ensures that enterprises remain agile and responsive, even as their data needs evolve.
- Data Security: In an era where data breaches are increasingly common, ensuring the security of sensitive information is paramount. Data fabrics offer robust security features, safeguarding data at every touchpoint. From encryption at rest and in transit to advanced access controls and monitoring, this architecture prioritizes data protection, giving businesses the confidence to operate in a digital-first world.
As the data landscape continues to evolve, solutions like data fabrics will be indispensable in ensuring that organizations can manage their data and harness it to its full potential.
Challenges in Implementing Data Fabrics
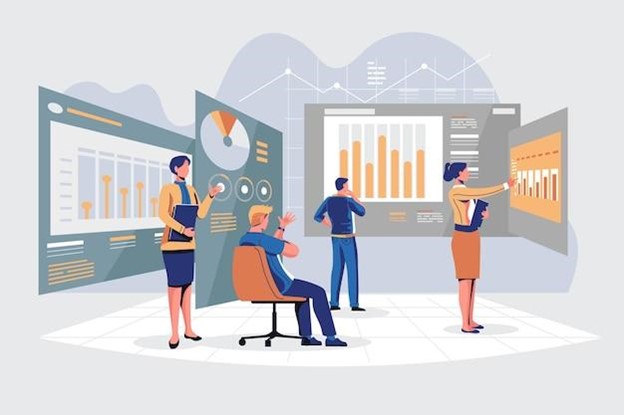
Image Source: freepik
While the data fabric architecture offers a transformative approach to data management, its implementation is not without challenges. As businesses embark on this journey, they often encounter a mix of technical, organizational, and structural obstacles. Recognizing and addressing these challenges is crucial for a successful transition to a data fabric architecture.
- Technical Challenges:
- Integration with Legacy Systems: Many enterprises still rely on older, legacy systems for their core operations. Integrating these systems with modern data fabric solutions can be complex, often requiring custom solutions and workarounds.
- Ensuring Data Quality: As the saying goes, “Garbage in, garbage out.” Ensuring the quality and accuracy of data is paramount. With data coming from multiple sources, maintaining its integrity becomes a significant challenge.
- Complexities of Integrating AI: While AI and machine learning offer immense potential in enhancing data fabrics, their integration is not straightforward. It requires expertise, the right tools, and a clear understanding of business objectives.
- Organizational Challenges:
- Change Management: Transitioning to a data fabric approach often requires a shift in organizational mindset. Managing this change, ensuring stakeholder buy-in, and navigating resistance can be challenging.
- Training: Data fabrics, with their features and capabilities, have a learning curve. Ensuring the workforce is adequately trained and equipped to use these tools effectively is vital.
- Fostering a Data-Driven Culture: Beyond just tools and technologies, a successful data fabric implementation requires fostering a culture where data-driven decision-making is the norm.
- Data Silos and Complexity:
- Addressing Data Fragmentation: In many organizations, data is scattered across various systems, databases, and platforms. These data silos hinder a unified view of information, making it challenging to derive meaningful insights.
- Unified Data View: With the data fabric’s promise of a unified data view, addressing the fragmentation and breaking down silos becomes crucial. It’s not just about integrating data; it’s about ensuring that this integrated data offers a holistic, 360-degree view, free from redundancies and inconsistencies.
In conclusion, while data fabrics present a promising solution to modern data challenges, their implementation requires careful planning, a clear strategy, and an understanding of the potential hurdles. By recognizing these challenges and proactively addressing them, businesses can ensure a smoother transition to data fabrics, unlocking their full potential.
Real-world Applications and Case Studies of Data Fabrics
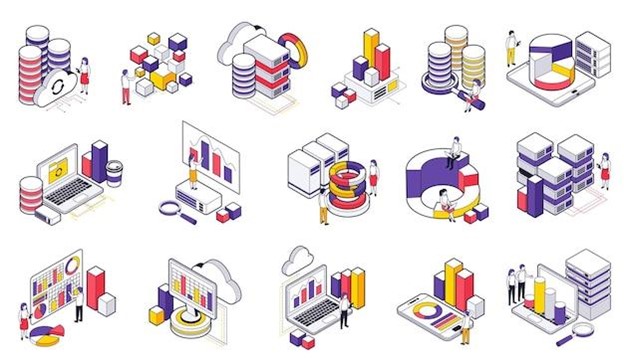
Image Source: freepik
Data fabrics’ transformative potential is actively reshaping industries, offering a more integrated, agile, and real-time approach to data management. Here’s a snapshot of its applications across various sectors:
- Healthcare:
-
- Patient Data Integration: Hospitals and clinics often use multiple systems to store patient data. Data fabrics can integrate these systems, providing healthcare professionals with a comprehensive view of a patient’s medical history.
- Research and Clinical Trials: Data fabrics can help in aggregating and analyzing data from various clinical trials, aiding researchers in drawing conclusions and making informed decisions.
- Supply Chain and Logistics:
-
- Inventory Management: By integrating data from suppliers, warehouses, and retailers, Data fabrics can provide real-time insights into inventory levels, helping businesses optimize stock levels and reduce costs.
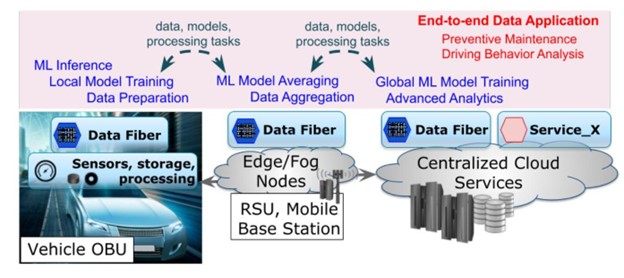
Intelligent Data Fabric for Connected Cars Applications by https://arxiv.org
-
- Route Optimization: For logistics companies, data fabrics can analyze data from various sources, such as traffic reports, weather forecasts, and vehicle telemetry, to suggest optimal delivery routes.
- Energy and Utilities:
-
- Smart Grid Management: Utilities can use data fabrics to integrate data from smart meters, grid equipment, and weather forecasts to optimize electricity distribution and predict equipment failures.
- Oil and Gas Exploration: Data fabrics can help energy companies integrate geological data from various sources, aiding in identifying potential drilling sites.
- Media and Entertainment:
-
- Content Recommendation: Streaming platforms can use data fabrics to integrate user preferences, viewing history, and content metadata to provide personalized content recommendations.
- Digital Rights Management: By integrating data from content creators, distributors, and consumers, data fabrics can help media companies track and manage digital rights, ensuring content is used appropriately.
- Finance:
-
- Efficient Liquidity Management: Financial institutions are leveraging data fabrics to forecast future cash flows. By integrating various data sources, they can proactively identify and plan for potential liquidity issues, ensuring optimal use of cash resources.
- Anti-money laundering (AML) Compliance: Compliance with AML regulations is paramount. Data fabrics aid in surfacing suspicious Know Your Customer (KYC) profiles and transaction information, providing a robust system to detect potential money laundering activities.
- Data-driven Scenario Planning: By integrating diverse data sources, financial institutions can discover new business opportunities, estimate the impact of various decisions, and bolster their decision-making processes.
- Streamlined Transaction Reconciliations: Reconciliation of transactions, especially in the face of reversed transactions, becomes more efficient. It aids in handling pending transactions and expediting the resolution of non-credit complaints.
- Retail and E-commerce:
-
- Conversion Anomaly Detection: Retailers can identify and investigate anomalies in conversion rates at various levels. This deep dive into data helps in understanding customer purchasing behavior, allowing businesses to optimize their strategies.
- Customer Behavioral Profiling: By integrating data from various touchpoints, retailers can gain profound insights into customer preferences. This aids in predicting demand, delivering targeted offers, and enhancing customer retention.
- Qualitative Survey Summarization: Data Fabric tools can extract critical insights from customer surveys, providing businesses with a qualitative summary of feedback on various factors, from product quality to customer support.
- Product Performance Insights: Retailers can analyze the performance of products across segments, uncovering trends and making data-driven decisions to optimize their offerings.
Data Fabrics and Modern Data Strategies in a Postmodern Era
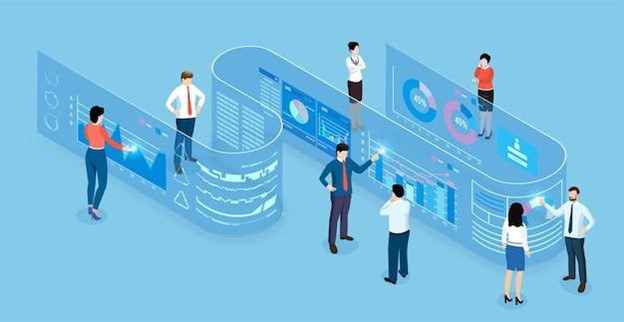
Image Source: freepik
In the rapidly changing world of data management, understanding current technologies is only half the battle. The real challenge lies in aligning these technologies with broader strategic paradigms. As we think about data fabrics, we must also consider its place within the postmodern data stack, a concept that’s reshaping the future of decision-making.
Data Mesh and the Postmodern Data Stack
The postmodern data stack is not just a set of tools; it’s a paradigm shift. It recognizes the need for agility in decision-making, especially in sectors like Fintech, where rapid growth demands quick insights. This approach emphasizes outcome-driven data science practices, shortening the time to build in-house decision-making products from months to mere weeks.
Data mesh, a decentralized data architecture, aligns perfectly with this vision. By shifting from centralized data lakes to domain-oriented, distributed data products, data mesh places data as a business-wide asset. When integrated with data fabric, which streamlines data from various sources, businesses can achieve a balance of agility and data integrity. This synergy allows organizations to harness the best of both worlds, driving innovation while maintaining data quality.
The cloud has revolutionized business operations, but with the advent of hybrid and multi-cloud environments, data management complexities have surged. Data fabrics act as a unifying layer, ensuring seamless data integration across these diverse environments. This is particularly crucial for Fintech companies, which need to harness data rapidly to gain key business insights.
Conclusion
The data landscape is in constant flux. As strategies like data mesh and cloud-centric approaches gain traction, the role of data fabrics becomes even more pivotal. In the postmodern era, it’s not just about managing data but harnessing its full potential.
In a world where data is the new oil, businesses cannot afford to be complacent. Embracing the data fabric architecture is not just a technological decision; it’s a strategic one. It’s about future-proofing your business, ensuring agility in the face of change, and maintaining a competitive edge in a data-driven world. As we stand at the cusp of a new era in data management, the question is not whether to adopt data fabrics, but how soon can you integrate it into your data strategy.