It’s the 24th century aboard the Starship Enterprise. Captain Jean-Luc Picard, in need of a break from the rigors of interstellar diplomacy, steps into the Holodeck. This isn’t just any room; it’s a technological marvel, a space where any scenario can be simulated, any world, any reality can come to life. Picard chooses a 1940s detective story, becoming Dixon Hill in a world of fedoras and trench coats. But a malfunction traps him and his crew in this simulated reality, turning a leisurely escape into a perilous adventure. Despite the unexpected danger, Picard uses the simulation to gain insights that later prove invaluable in real-world negotiations with a hostile alien race.
Modern-day enterprises have their own version of a Holodeck, albeit less cinematic but equally transformative—generative AI. This new-age technology can simulate business scenarios, market trends, and customer behaviors with astonishing accuracy. And much like Picard’s adventure, it’s not without its risks and malfunctions. But when navigated wisely, it offers invaluable insights that can shape the future of an entire organization.
The Mechanics of Generative AI
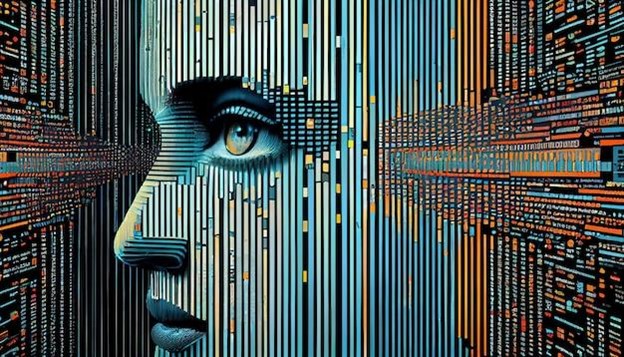
Source: Freepik
Generative Adversarial Networks, or GANs, have revolutionized the AI landscape. Picture a duet between two musicians: one crafts the melody, and the other critiques it. The generator, like the composer, creates new data instances, while the discriminator, the critic, evaluates them. This dynamic duo works in a feedback loop. The generator takes the discriminator’s feedback to refine its next masterpiece. Over iterations, the fenerator becomes so adept that its creations are virtually indistinguishable from real-world data. This prowess has made GANs a cornerstone in enterprise analytics, where synthetic yet realistic data is invaluable for training robust machine learning models.
Other Generative Models
While GANs often steal the show, other generative models like Variational Autoencoders (VAEs) and Restricted Boltzmann Machines (RBMs) also deserve the spotlight. VAEs are the detectives of the data world, excelling at uncovering the hidden structures within data. They shine in tasks like anomaly detection, where discerning the subtleties of ‘normal’ data is crucial. RBMs, the Swiss Army knives of generative models, have a wide range of applications, from recommendation systems to feature reduction.
The Challenge of Accuracy in Open-Ended Interactions
Navigating the labyrinthine complexity of generative models brings us to a critical juncture: the imperative of accuracy, especially in open-ended interactions. In the high-stakes arena of enterprise analytics, a slight misstep can have significant repercussions. Whether it’s a customer service bot misunderstanding a query or a predictive model inaccurately simulating market trends, the margin for error is slim. The quest for accuracy is not merely a technical hurdle; it’s a business necessity. Fortunately, the field is making strides, with research continually pushing the boundaries of what’s possible.
Applications of Generative AI in Enterprise Analytics
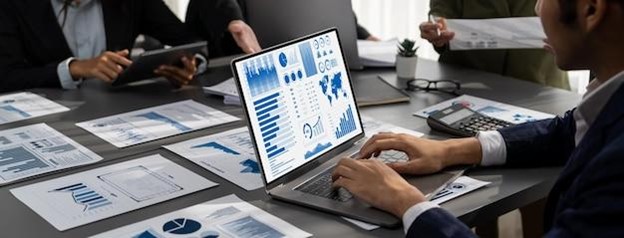
Source: Freepik
In the enterprise world, the user interface is more than a mere point of entry; it’s a vital part of the user experience. Generative AI platforms are revolutionizing this space by enabling natural language interfaces that adapt to user behavior and preferences. Platforms like Salesforce’s Einstein Analytics and Scribble Data’s Hasper allow data analysts to query, “Show me the sales trends for the last quarter,” and the AI translates this into an SQL command, fetching the relevant data. This intuitive interaction democratizes analytics, making it accessible and user-friendly. IBM’s Watson Assistant offers a similar conversational interface for businesses, streamlining customer interactions and internal processes. These platforms are not just making life easier for data analysts; they’re transforming the way businesses interact with data.
Nudges and the Humanization of Data
Generative AI platforms are particularly adept at producing data that mimics human behavior and preferences. In healthcare, GPT-based models have been used to generate personalized follow-up messages for patients, making them feel more engaged and cared for. This isn’t merely about making data relatable; it’s about enriching analytics by adding a layer of human understanding. In the realm of e-commerce, Amazon uses generative AI models to create personalized shopping experiences, nudging users toward products they are likely to purchase. The AI-generated nudges are so subtle and personalized that they feel almost human.
Data Augmentation
Data is the cornerstone of analytics, and generative AI platforms can synthesize datasets that mirror real-world conditions. For instance, in the field of autonomous vehicle development, Waymo and Tesla use generative AI models to create synthetic datasets that simulate various driving conditions. This enables more robust model training and, consequently, safer autonomous vehicles. Similarly, in agriculture, the use of generative AI models to simulate crop growth under different environmental conditions is being explored, aiding in the development of more resilient crops.
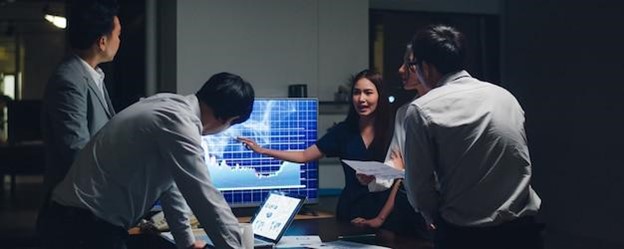
Source: Freepik
Predictive Modeling
Predictive models have always been valuable tools, but generative AI platforms take this a step further by simulating entire scenarios. Financial institutions like JPMorgan Chase are increasingly using generative AI to simulate economic conditions under various scenarios, helping them prepare for different market conditions. It’s not merely about forecasting an outcome; it’s about comprehending the spectrum of possibilities and preparing for them.
Personalization and Automated Insights
Personalization is no longer a luxury; it’s a necessity. Generative AI platforms adapt user experiences based on generated content. Netflix, for example, uses generative AI to create personalized recommendations, enhancing viewer engagement. Moreover, these platforms can automatically derive insights from data, offering personalized solutions to business problems. This is like having a custom-tailored suit—designed to fit, impress, and meet your unique needs, all thanks to algorithms that understand your business.
Real-world Implementations of Generative AI
While we’ve delved into the transformative potential of generative AI in enterprise analytics, it’s crucial to recognize that its applications extend far beyond that realm. From healthcare and manufacturing to entertainment and retail, generative AI is making waves across various industries. Let’s explore some of these real-world implementations that are not only innovative but are also setting new benchmarks for what technology can achieve.
Healthcare: A New Frontier in Drug Discovery and Personalized Medicine
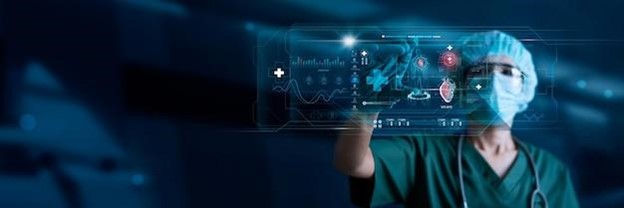
Source: Freepik
In the healthcare sector, generative AI is revolutionizing drug discovery and personalized medicine. Take the example of Insilico Medicine, a company that utilizes generative AI to accelerate the drug discovery process. By generating molecular structures that could potentially be new drugs, they’ve managed to cut down years of manual research into a matter of months. This is not just a technological marvel; it’s a life-saving innovation.
Manufacturing and Supply Chain: The Optimization Game
When it comes to manufacturing and supply chain logistics, generative AI is a game-changer. BMW, for instance, uses generative AI algorithms to optimize its manufacturing processes. These algorithms can simulate countless scenarios to find the most efficient way to utilize resources, from raw materials to human labor. Similarly, Amazon employs generative AI in its supply chain to predict and manage inventory levels, thereby reducing costs and improving customer satisfaction.
Entertainment and Media
The entertainment and media industry is another sector where generative AI is making significant strides. NVIDIA, a name synonymous with cutting-edge technology, uses generative AI to create hyper-realistic environments for video games. These aren’t your run-of-the-mill backgrounds; they’re dynamic ecosystems that react and evolve, offering gamers an immersive experience like never before.
Retail and Customer Experience: The Personal Touch
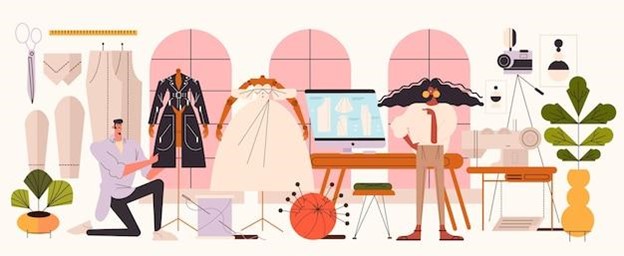
Source: Freepik
In the retail sector, generative AI is all about enhancing customer experience. Online styling services use generative AI to curate personalized fashion choices for their customers. The AI model analyzes customer data, fashion trends, and even textile information to generate style recommendations that are as unique as the individual receiving them. Similarly, Sephora employs generative AI models to offer virtual try-ons for its makeup products, allowing customers to see how different products will look on them without ever stepping into a store.
This is just the tip of the iceberg; as the technology matures, its potential applications are bound to expand, offering unprecedented opportunities for innovation and growth.
The Future of Generative AI in Enterprise Analytics
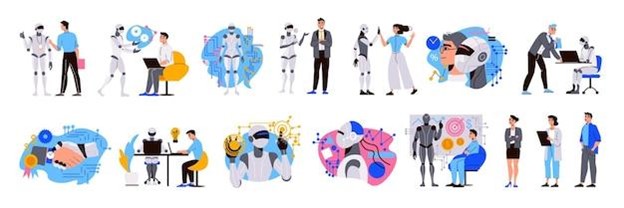
Source: Freepik
Widespread Deployment
The future of generative AI in enterprise analytics is not a distant dream; it’s unfolding right before our eyes. As we speak, companies are moving beyond pilot projects and isolated use cases to full-scale deployment. The goal is to integrate generative AI models into the very fabric of business operations. Imagine a world where your supply chain is not just efficient but self-optimizing, thanks to generative algorithms that continuously learn and adapt. It’s not science fiction; it’s the next logical step in enterprise evolution.
More Precomputation and Recommendations
As generative AI technology matures, we’re likely to see a shift towards more precomputation and automated recommendations. Instead of merely generating data or simulating scenarios, future generative AI platforms will offer actionable insights. Think of it as your analytics department having a virtual round-table discussion with AI, where the AI not only brings data to the table but also interprets it and suggests strategies. The focus will shift from “What does the data say?” to “What should we do about it?”
De-emphasis of Dashboards
Dashboards have been the go-to for data visualization and quick insights. However, as generative AI becomes more integrated into analytics processes, the reliance on dashboards will diminish. The future lies in intuitive, natural language interfaces and real-time alerts that provide insights as and when they are needed, without the need to sift through complex dashboards. It’s similar to having a personal analytics assistant that knows what you need before you even ask.
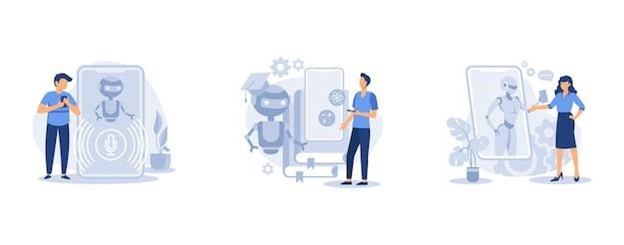
Source: Freepik
New Roles Involving Managing the AI
As generative AI takes on more responsibilities, new roles will emerge within organizations. These roles won’t be about managing data but about managing the AI itself. Companies will need experts who can fine-tune generative models, interpret their outputs, and integrate these insights into business strategies. It’s not just about having AI skills; it’s about having the business acumen to translate AI capabilities into competitive advantages.
The enterprises that recognize this potential and invest in generative AI platforms now will be the trailblazers of tomorrow, setting new standards for innovation, efficiency, and strategic foresight.
Challenges and Ethical Considerations
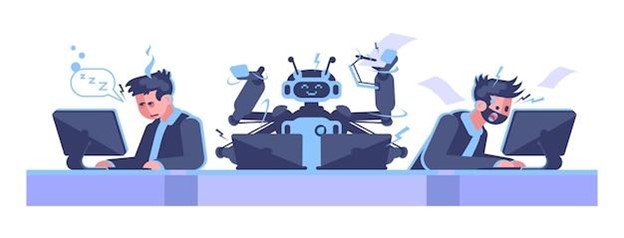
Source: Freepik
The Fine Line Between Generated and Manipulated Data
As we venture deeper into the realm of generative AI, the boundaries between generated and manipulated data become increasingly blurred. While the technology offers unprecedented capabilities for data augmentation and predictive modeling, it also raises questions about data integrity. For instance, in the financial sector, the use of generative AI to simulate market trends could inadvertently lead to market manipulation if not carefully regulated. It’s a double-edged sword that demands vigilant oversight.
Ethical Concerns About Generating Misleading Information
The ethical implications of generative AI are far-reaching. Consider the healthcare sector, where generative AI is used for drug discovery. While the technology can accelerate the development of life-saving medications, what happens if it generates a compound that, although effective in simulations, proves harmful in real-world applications? The stakes are high, and the ethical burden is immense. It’s not just about generating data; it’s about the ramifications of that data on human lives and societal structures.
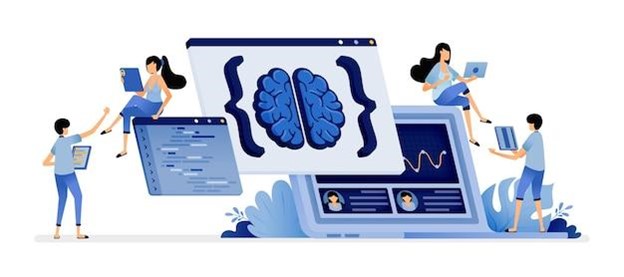
Source: Freepik
Ethical considerations are not an afterthought; they are integral to the development and deployment of generative AI. Experts in the field are actively engaged in establishing guidelines and frameworks to ensure that the technology is used responsibly. This includes measures to prevent the amplification of biases and the potential for making wrong decisions based on generated data.
Technical Challenges in Implementing and Scaling Generative AI Solutions
Implementing generative AI is not without its technical hurdles. The computational resources required for training sophisticated generative models can be prohibitive for smaller enterprises. Additionally, the complexity of these models makes them prone to errors and inaccuracies, requiring constant fine-tuning and oversight. As we scale these solutions, the challenges multiply, from data security concerns to the need for specialized talent to manage and interpret the AI’s outputs.
In navigating these ethical and technical challenges, a responsible AI strategy becomes not just an option but a necessity. Organizations must implement frameworks that govern the ethical use of AI, ensuring that it aligns with broader societal values and norms. A responsible AI strategy serves as a roadmap for ethical decision-making, guiding enterprises in the deployment and management of generative AI technologies.
Misconceptions and Realities about Generative AI
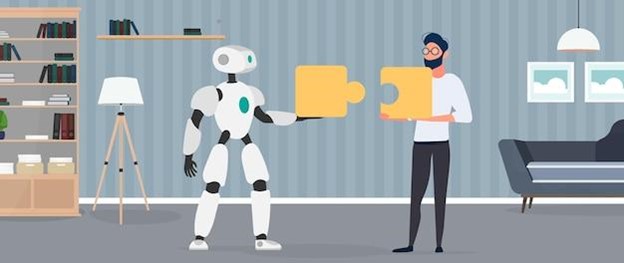
Source: Freepik
The Myth of Job Replacement
One of the most pervasive myths surrounding generative AI technology is the fear of job replacement. The narrative often goes like this: “If machines can generate data, write code, and even simulate human-like interactions, what’s left for us?” The reality, however, is far from this dystopian vision. Generative AI is designed to augment human capabilities, not replace them. It takes over mundane tasks, freeing up human resources to focus on more complex, creative, and emotionally nuanced work.
The Reality Check: Systems and Training
While generative AI models offer a plethora of advantages, it’s not a plug-and-play solution. Effective deployment requires a well-thought-out system architecture and ongoing training for staff. The technology is only as good as the human expertise guiding it. For instance, a generative AI system used in healthcare for drug discovery needs to be calibrated and overseen by experts in pharmacology and medicine.
The Amplification of Roles
Generative AI platforms don’t just take away tasks; they add new dimensions to existing roles. For example, data analysts equipped with generative AI tools can move beyond mere number-crunching to become strategic advisors. They can interpret complex data patterns, offer insights, and even predict future trends, thereby amplifying their role within the organization.
The Importance of Public Perception
Lastly, the way generative AI is perceived by the public plays a crucial role in its adoption and effectiveness. Misconceptions can lead to unwarranted fears and resistance. Therefore, transparent communication about the capabilities, limitations, and ethical considerations of generative AI is vital for its successful integration into society.
Understanding the myths and facts surrounding the use of generative AI is key to leveraging its full potential. While it’s not the job-stealing monster some make it out to be, it’s also not a magical solution that works right out of the box.
The Future is Bright, But Not Without Its Challenges
One of the most exciting future trajectories for generative AI in enterprise analytics is the gradual shift from static dashboards to dynamic decision rooms. Imagine a virtual space where real-time data is not just displayed but interactively manipulated, allowing for instantaneous scenario planning and decision-making. As generative AI continues to mature, we may also see the emergence of new roles within organizations.
Generative AI-powered analytics is not a passing trend; it’s a transformative technology that is here to stay. By understanding its mechanics, applications, real-world implementations, and the myths that surround it, we can prepare ourselves for a future that is as exciting as it is unpredictable.